Recurrent Neural Network (RNN) and Long Short-Term Memory (LSTM) network are useful tools for modeling sequential data.
RNN
Starting from input. It is time-dependent. Hence, denoted by $$ \boldsymbol{x}_t $$ where $\boldsymbol{x}_{t}\in\mathbb R^{n}$, $t=1,2,\ldots, T$.
Formulation 1
On output, one fashion is a single output $\boldsymbol{y} \in \mathbb R^{m}$ (at the final time step $T$), that is to say: $$ f(\{\boldsymbol{x}_{t}\}) = \boldsymbol{y} $$ This can be useful in sentiment analysis.
- This seems to be a problem even approachable by regression or classification.
- But notice that the input dimension can be large ($n\times T$), or even not fixed length (since $T$ is sample-dependent). Although truncation or padding can be applied, but still, it seems to be suboptimal to model like this.
Formulation 2
Another way to model this problem is that we seek a function that predicts a $\boldsymbol{y}_{t}$ for each $\boldsymbol{x}_{t}$, that is to say, $$ f(\boldsymbol{x}_{t}) = \boldsymbol{y}_t $$ and we only take the final outcome $\boldsymbol{y}_{T}$ as the targeted outcome. The progresses here are:
- Input dimension has been reduced (from $n \times T$ to $n$), which is not sample-dependent anymore!
- The downside for this formulation is that there is no memory of the previous information at all.
- Output at each step is also interesting in translation or language generation task, where we care about all the outputs $\{\boldsymbol{y}_{t}\}$.
- This is also related in
return_sequence
option in Keras.
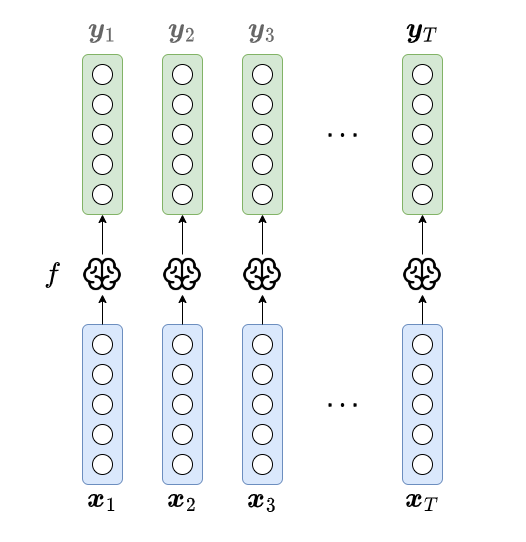
Formulation 3
To add dependency, we can formulate the model as $$ f(\boldsymbol{x}_{t}, \boldsymbol{y}_{t-1}) = \boldsymbol{y}_{t}, \qquad t=1,2,\ldots, T. $$ with inital condition of $\boldsymbol{y}_{0}$ (set as $\boldsymbol{0}$ or something else).
- This formulation includes (immediate) short-term memory explicitly.
- The input dimension becomes $n + m$, slightly larger than Formulation 2.
- The output $\boldsymbol{y}_{t}$ is sometimes called hidden states (denoted as $\boldsymbol{h}_{t}$) in some context. Sometimes, there will be another $\tanh$ transformation applied, sometimes, it is just a copy of $\boldsymbol{h}_{t}$. It is especially so in the context of LSTM.
- However, RNN suffers from a problem called lack of long-term dependency (in practice, not theoretically). (Though "Why we need long term memory?" is still arguable.)
- The number of output is the same as the number of inputs, which is probably not flexible enough.
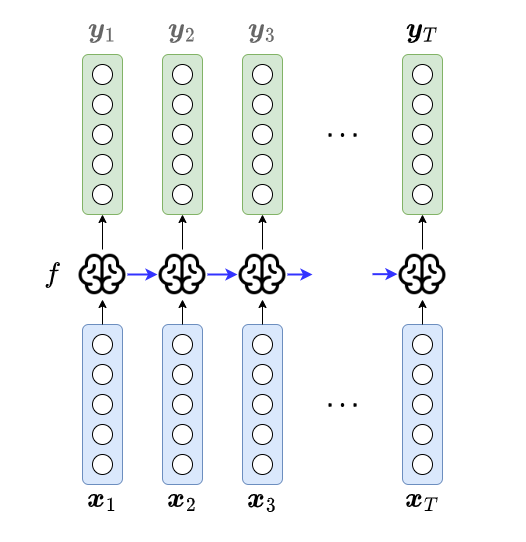
Formulation 4 (seq2seq)
To really get one step closer to translation or conversational robots, seq2seq proposed by Mikolov is one milestone. Basically, it is a combination of formulation 1 (encoding — to understand the input) and 2 (decoding — to generate output).
LSTM
Why LSTM? — Major benefit: long-term memory.
Major contributions
Cell state: $\mathbf c_{t}$. (a.k.a. carry state as commented in Keras source code.)
- Why? keep "encoded" long-term memory.
- Key point: only linear transformation applied to this cell.
- Dimension: $\mathbb R^{n}$.
Gates:
- Including: forget gate ($\mathbf f_{t}$), input gate ($\mathbf i_{t}$), output gate ($\mathbf o_{t}$).
- Dimension: $\mathbb R^{n}$.
Steps
Put in language:
- Compute forget gate (to forget some past memory)
- Compute input gate (to digest some new memory)
- Compute output gate (be mindful on what to output)
- Compute candidate memory
- Update memory by
- Compute output
Formula-form: $$ \begin{aligned} \mathbf{f}_{t} &= \sigma( \mathbf{W}_{\mathbf{f}} [\mathbf{h}_{t-1}, \boldsymbol{x}_{t}])\\ \mathbf{i}_{t} &= \sigma( \mathbf{W}_{\mathbf{i}} [\mathbf{h}_{t-1}, \boldsymbol{x}_{t}])\\ \mathbf{o}_{t} &= \sigma( \mathbf{W}_{\mathbf{i}} [\mathbf{h}_{t-1}, \boldsymbol{x}_{t}])\\ \widetilde{\mathbf{c}}_{t} &= \tanh(\mathbf{W}_{\mathbf{c}} [\mathbf{h}_{t-1}, \boldsymbol{x}_{t}])\\ \mathbf{c}_{t} &= \mathbf{f}_{t} \odot \mathbf{c}_{t-1} + \mathbf{i} \odot \widetilde{\mathbf{c}}_{t}\\ \mathbf h_{t} &= \mathbf{o}_{t} \odot \tanh(\mathbf{c}_{t}) \end{aligned} $$
Variants
Peephole connections
If we want memory has a say at the gate:
- Compute forget gate by $\mathbf{f}_{t} = \sigma( \mathbf{W}_{\mathbf{f}} [\textcolor{DodgerBlue}{\mathbf{c}_{t-1},} \mathbf{h}_{t-1}, \boldsymbol{x}_{t}])$
- Compute input gate by $\mathbf{i}_{t} = \sigma( \mathbf{W}_{\mathbf{i}} [\textcolor{DodgerBlue}{\mathbf{c}_{t-1},} \mathbf{h}_{t-1}, \boldsymbol{x}_{t}])$
- Compute output gate by $\mathbf{o}_{t} = \sigma( \mathbf{W}_{\mathbf{o}} [\textcolor{DodgerBlue}{\mathbf{c}_{t},} \mathbf{h}_{t-1}, \boldsymbol{x}_{t}])$
Questions:
- Why not $\mathbf{c}_{t-1}$ in output gate?
Coupled forget and input
Update step 5 by
- Update memory by $\mathbf{c}_{t} = \mathbf{f}_{t} \odot \mathbf{c}_{t-1} + \textcolor{DodgerBlue}{(1-\mathbf{f}_{t})} \odot \widetilde{\mathbf{c}}_{t}$
Also, delete step 2.
Gated Recurrent Unit (GRU)
On a high level GRU does the following: $$ \begin{aligned} \text{forget gate} + \text{input gate} &= \text{update gate} (\mathbf{z}_{t})\\ \text{cell state} + \text{hidden state} &\rightarrow \text{hidden state} \end{aligned} $$ Formula-wise: $$ \begin{aligned} \mathbf{z}_{t} &= \sigma(\mathbf{W}_{\mathbf{z}}[\mathbf{h}_{t-1},\boldsymbol{x}_{t}])\\ \mathbf{r}_{t} &= \sigma(\mathbf{W}_{\mathbf{r}}[\mathbf{h}_{t-1},\boldsymbol{x}_{t}])\\ \widetilde{\mathbf{h}}_{t} &= \tanh(\mathbf{W}_{\mathbf{h}}[\mathbf{r}_{t} \odot \mathbf{h}_{t-1}, \boldsymbol{x}_{t}])\\ \mathbf{h}_{t} &= (1-\mathbf{z}_{t}) \odot \mathbf{h}_{t-1} + \mathbf{z}_{t} \odot \widetilde{\mathbf{h}}_{t} \end{aligned} $$
Attention $\rightarrow$ Transformer
There is a pretty fancy exhibition of how the attention mechaism works by Google AI blog post:
The high level idea is to utilize better historical information.
Troubleshooting: Gradient Vanishing and Explosion
How to Fix Vanishing?
- Use ReLU
- batch normalization
- ResNet
- weight initialization
How to Fix Explosion?
- Re-design your model using fewer layers
- smaller batch size
- truncated backprop through time
- use LSTM
- use gradient clipping
- use weight regularization
- batch normalization
Other Literatures and Future Reading
- Depth Gated RNN — Yao (2015)
- Clockwork RNN — Koutnik, et al. (2014)
- Grid LSTM — Kalchbrenner, et al. (2015)
- Generative models — Gregor, et al. (2015), Chung, et al. (2015), Bayeer & Osendorfer (2015)
- Review paper — Greff, et al. (2015); Jozefowicz, et al. (2015)
- Bidirectional LSTM
- Bidirectional Encoder Representation from Transformer (BERT)
- Generative Pre-trained Transformer 3 (GPT-3)
- Chat Generative Pre-trained Transformer (ChatGPT)